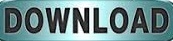
A amostra conseguida é composta por 30 empresas de médio e grande portes. Aplicou-se questionários estruturados e foram realizadas entrevistas em profundidade com especialistas para obtenção de dados e informações. A pesquisa descrita neste artigo buscou identificar o grau de implementação do PE e TE e, ainda, avalia o seu impacto nos processos de controle e melhoria e a satisfação com essa implementação nas empresas do setor químico localizadas no estado de São Paulo, Brasil. The relevance of the method is emphasized by an application to a real case study related to the assembly of rotating ring wrapping machines used in end-of-line packaging.Īs abordagens para a gestão da produção e melhoria são baseadas em tomadas de decisão baseadas em fatos e auxiliadas pelo Pensamento Estatístico (PE) e pelo uso de Técnicas Estatísticas (TE). Detecting critical workstations facilitates quality engineers in identifying the causes of non-conformities and undertaking appropriate corrective actions.
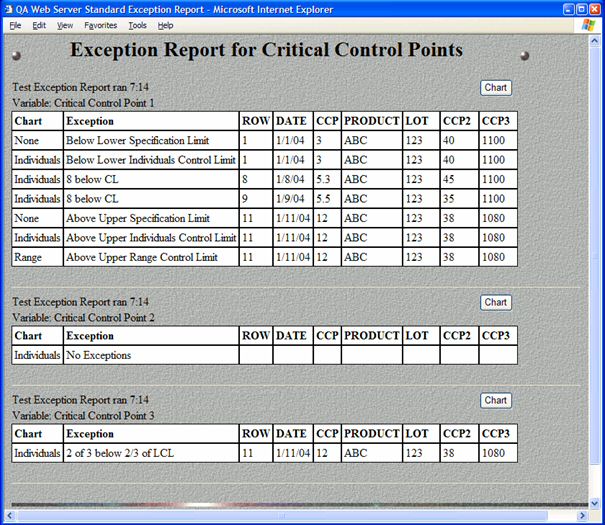
The methodology identifies critical assembly workstations where the respective average defectiveness deviates from the average defectiveness predicted by the model. Assembly complexity of both the process and the design are used as predictors of the defect model. The method is based on a self-adaptive defect prediction model of the process, updated as new data are acquired. This paper proposes a diagnostic tool that provides an in-line identification of critical steps of assembly processes. In low-volume productions, characterized by a high level of complexity and customization, defects prediction may be challenging owing to the small amount of historical data typically available. The prediction of defects occurring during manufacturing processes is one of the strategies to be implemented by organizations to reach the goals of Zero-Defect Manufacturing (ZDM). The failure modes and effect analysis was used to identify and eliminate sources of variation, which led to a reduction in process variation by 63 per cent and a decrease in defects by 160 961 per million opportunities. The diagnostic ability of statistics, coupled with a failure modes and effect analysis (FMEA) as the problem-solving methodology during an out-of-control action plan, creates an environment conducive to improving processes and empowering employees. However, SPC can only be effective if implemented with a structured problem-solving tool. Statistical process control (SPC) and statistical quality control have been widely used in quality management to enhance process performance by reducing process variation. The ultimate aim remains customer satisfaction, using the most effective techniques to ensure the most efficient and cost-effective process. It is now a significant component of holistic operational management. Quality management has ceased to be an operational extra, and has become imperative to doing business in a saturated market environment with demanding clients. An example of implementation of the method in wrapping machines production is presented and discussed. Once the causes leading to significant nonconformities have been detected, appropriate corrective actions may be promptly undertaken to improve the process.
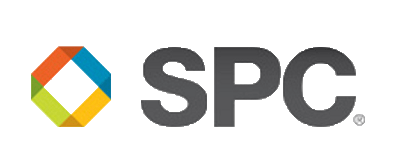
Starting from the level of complexity in terms of assembly process and design, the method allows identifying the workstations whose defectiveness deviates, at a certain confidence level, from the predicted average value. This paper proposes an innovative method for identifying critical workstations in assembly processes based on defect prediction models. Accordingly, identifying effective diagnostic tools plays a key role in such productions. As a consequence, the scarcity of historical data and the difficulty in applying standard statistical techniques make process control extremely challenging. Assembly processes in low-volume productions, i.e., single-units or small-sized-lots, are often characterized by a high level of customization and complexity.
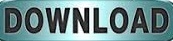